In today's data-driven world, the ability to efficiently ingest and process data is a cornerstone of successful AI/ML workflows. It is a critical step because the quality and efficiency of data ingestion directly impacts the accuracy, performance and scalability of reliable AI models that are developed using trustworthy data. In this blog, we'll explore how leveraging NetApp SnapMirror and JupyterHub can simplify and enhance the data ingestion process for AI/ML workflows. We'll provide an overview of the key concepts and processes, and for those interested in a deeper dive, our supplemental video covers the topic in detail.
Effective data ingestion for AI/ML workflows is crucial for several reasons:
- Real-time Insight: Ensures AI-ML models have up-to-date data for accurate predictions.
- Scalability: Supports growth by preventing performance bottlenecks as data volumes increase.
- Consistency: Guarantees accurate and consistent data transfer from various sources.
- Data Readiness: Reduces data preparation time and effort.
Using NetApp BlueXP for Simplified SnapMirror Setup:
To facilitate efficient data ingestion, we turn to NetApp BlueXP. This tool offers an intuitive interface for setting up NetApp SnapMirror, making the data replication process straightforward. By utilizing SnapMirror, we can seamlessly replicate data from a source data center to our AI-ML processing hub. Following is the architecture and key steps for Data Ingestion:
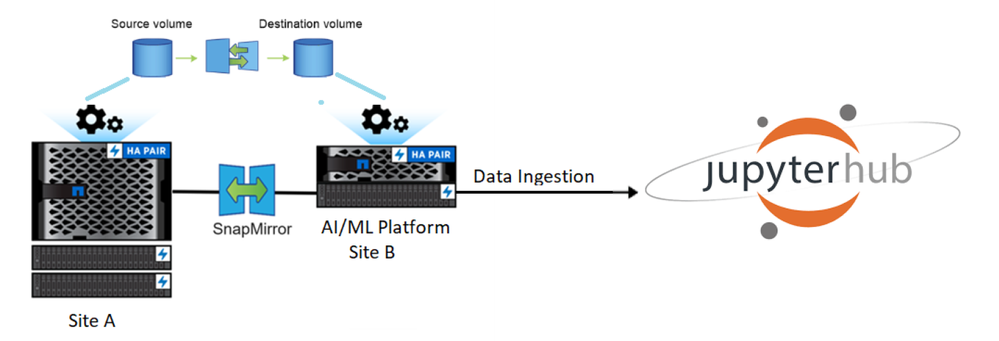
-
Initiating the SnapMirror Relationship: With NetApp BlueXP, setting up replication is as simple as dragging the source cluster icon onto the destination cluster. By selecting the appropriate volume and specifying necessary details, we can easily start the replication process.
-
Creating a Clone for Experimentation: Once data is replicated, a clone of the destination volume is created. This clone will be used within JupyterHub, allowing data scientists to access and run their experiments on the replicated data.
-
Mounting the Clone in JupyterHub: The final step involves integrating the cloned volume into JupyterHub. This makes the data readily available for AI-ML models, enabling seamless experimentation and analysis. We can do this without bringing down the JupyterHub environment and disrupting active users.
For a detailed walkthrough of this data Ingestion exercise you can follow along with the video below: